If you are new here, welcome! Alfred Intelligence is my personal notes as I learn to be comfortable working on AI. Check out the first issue of Alfred Intelligence to understand why I started it.
Many people have said 2025 will be the year of agents. Sam Altman, perhaps one of the most credible sources, wrote in his latest blog post:
We believe that, in 2025, we may see the first AI agents “join the workforce” and materially change the output of companies.
But what would AI agents actually look like? What should AI agents look like? Or more precisely, what should working with AI agents look like?
This week, I spent much of my time looking at existing AI agents and pondering about the possible user interfaces and experiences for AI agents. Here are my notes:
How much information to show, and how to show it
This is one of the interesting design challenges that came up this week.
To build trust in the AI agent, we might want to show as much information as possible, all the agent’s thought processes, actions, and reflections. This way, the user knows exactly what the agent is thinking and doing. Claude computer use does this. It streams all the thought processes, actions, and screenshots in the chat interface.
But this quickly overwhelms the user with too much information. So many messages are displayed that it is hard for the user to find the important ones.
On the other hand, you could argue that since agents are meant to be autonomous, like remote teammates, we don’t have to know everything they did, as long as they produce the desired result. For example, in Otto, each spreadsheet cell is an agent that goes off and searches the web or analyzes your file for you. It simply returns the result (and its confidence in the result) in the cell, without showing its thoughts and steps.
But this has two issues:
Finding information on the web is a relatively simple task. This interface might not work for multi-step tasks because if it fails, we would want to know what went wrong and where.
Agents are much more likely to make a mistake for multi-step tasks because their error rate compounds. A 95% success rate for a one-step task becomes 60% for a ten-step task.
One of the better implementations I have seen is BabyAGI-UI by Yoshiki Miura. Instead of showing every single thought and step by the agent, it has a block for each task in the agent-generated task list. If I want more details, I can expand the block to see the things the agent did and a summary of that particular task.
Perplexity has a similar interface, which shows its steps with a toggle to see more details. But some steps displayed only a progress bar or “Working (checkmarks)” without much information. Upon reflection, this seems fine because I got the result I was looking for and I didn’t care how it completed that step. Perhaps for research in general, this is acceptable as long as the sources are listed.
But as Jet New pointed out, this works well only for a linear work process. In the ideal world, an agent should do things in parallel when possible. This linear interface will not be suitable for displaying such a work process. Also, if the agent fails to get the desired result for an intermediate step, it might change its plan and try something else. How do we show the work process with the various attempts? Or is it necessary to show all the attempts? If it is, perhaps tree or graph representation will be better.
Another good implementation is by Devin. I can ping it in Slack, a tool that is always kept open on my computer and available on my phone. I can interact with it on Slack, and it will go off and work on the task somewhere else. It will share a link with me to the Devin web app, where I can follow the progress if I want.
The web app shows several things that Devin interacts with for each task: the user (via chat), the browser, the terminal, and the code. How much should it show? Users can switch between a two-panel display and a four-panel display. The former displays two panels that are large enough to work with but I’m required to switch between a few tabs under Devin’s Workspace. The latter is great for showing all four “mini-apps” but each could be too small to be useful. It’s a tough balance! There also seems to be a slider where I can adjust and see the progress at each step1.
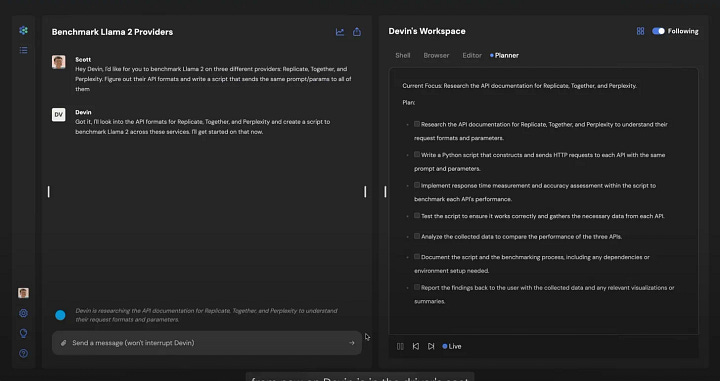
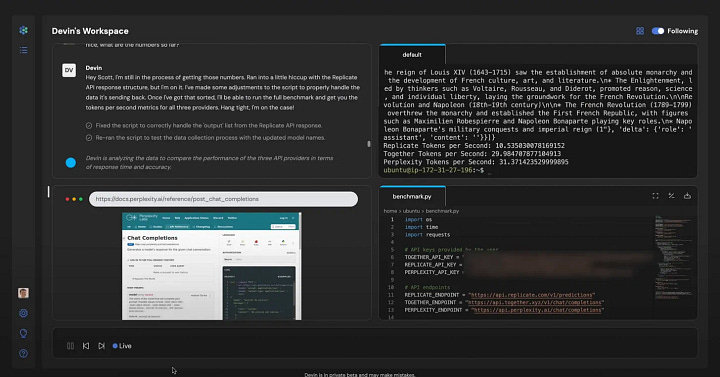
Here’s another well-design interaction: When it completes the task, it will create a pull request on GitHub for me to review before I approve the changes. This prevents it from changing my code in ways that I do not want or that could cause issues. But this works well with code, which has git, and not for other documents (unless we use something like Lix) or posting on Instagram or sending an email. In the latter scenarios, the agent should ask for confirmation, unless the user doesn’t feel the need.
That last point raises another important design question: When should the agent ask for permission before taking an action?
Again, the ideal goal is to let the agent do things for us autonomously. If we need to approve every step along the way, it defeats the point. But, given agents have a relatively high chance of making mistakes, we might want to prevent them from making irreversible drastic mistakes. Sending an angry email to our boss. Posting personal information on Instagram. Commenting sensitive information on Reddit. Perhaps we could get the agent to decide when to get our approval and when an approval isn’t necessary. However, there is the risk of compounding another mistake. We could even use another large-language model (LLM) to judge and improve the decisions of the agents (this is often known as “AI judge”).
I’ll be thinking a lot more about human-agent interfaces and interactions these few weeks. I’ll be happy to report back when I have more ideas. Let me know what you think about this too!
Jargon explained
WebVoyager: Last week, we learned about the OSWorld benchmark, a test of 369 handpicked real-world computer tasks for AI agents. This week, I came across WebVoyager. It is a web agent for complex web tasks on real-world websites. The researchers tested it with 643 tasks, which eventually became known as the WebVoyager benchmark, for testing web agents.
Chain of Thought (CoT) and ReAct: These are terms you cannot avoid when learning about AI agents. They are different ways to prompt the model to get better results. CoT is asking the model to think step by step, such as appending “Please explain step-by-step.” after your question. ReAct is asking the model to reason, act, and reflect. You can either describe this in the prompt (example below) or use a framework like LangChain.
You are an AI assistant that uses reasoning and actions to solve problems step-by-step. Follow this structure:
- **Thought**: Think about what you need to do first.
- **Action**: Describe what action you would take (like searching, calculating, etc.).
- **Observation**: Imagine the result of your action.
- Repeat until the task is complete.
- **Conclusion**: Summarize the final answer.
Now, solve this question using the structure above:
"How many countries are in Africa, and which one has the largest population?"
The main difference is that when you use ReAct, you also need to give the model access to tools, APIs, or data sources so that it can act. You can see my full ChatGPT conversation with more details and examples here.
DeepSeek-V3: DeepSeek-V3 was launched last month and made the news because it outperformed Llama 3.1 and Qwen 2.5 and matched GPT-4o and Claude 3.5 Sonnet while being really cheap. Its API, even without the current discount, costs only $0.07/1M input tokens and $1.10/1M output tokens. In comparison, GPT-4o costs $2.50/1M input tokens (35x more expensive) and $10.00/1M output tokens (9x more expensive). DeepSeek’s API price is closer to that of Gemini 1.5 Flash.
Interesting links
Agents: This is an easy-to-read introduction to AI agents, even if you only roughly know LLMs and coding.
Agentive Tech and Agentic AI: Cousins not adversaries: Chris Noessel wrote Designing Agentive Technology: AI That Works for People in 2017(!!). In this article, he listed new design considerations, given the new abilities of LLMs.
UX for Agents, Part 1, 2, 3: LangChain cofounder and CEO, Harrison Chase wrote about various possible agent experiences, including chat, ambient, spreadsheet, generative, and collaborative UX.
Recent issues
If you notice I misunderstood something, please let me know (politely). And feel free to share interesting articles and videos with me. It’ll be great to learn together!
I have not tried Devin, but I inferred this based on their website and videos.